Correlation vs. Causation and the Challenge of “Sorta” Statistics in Determining Coverage Rates
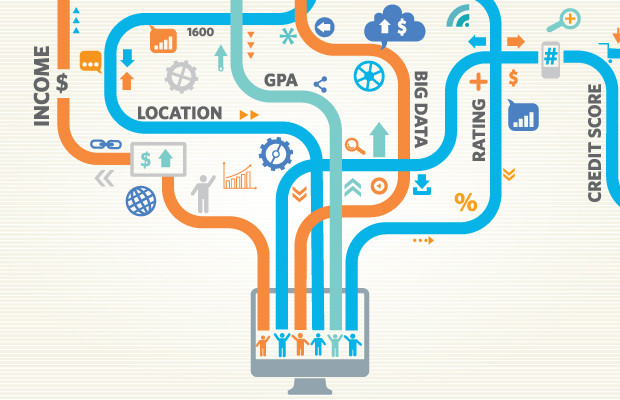
In a popular 2015 Super Bowl commercial , a woman walks into her local pharmacy to pick up a prescription and is awkwardly greeted by AMC’s Breaking Bad anti-hero Walker White. Confused about why her pharmacist “Greg” isn’t behind the counter, the TV show’s high-school-chemistry-teacher-gone-criminal-mastermind replies, “I’m sorta Greg.”
The commercial is among others in the ad campaign of a leading car insurance company that takes a comical look at the statistics used to determine rating factors. White continues with his Greg comparisons: “We’re both over 50 years old…we both have a lot of experience with drugs…sorry, pharmaceuticals.”
Predictive analytics has been an effective tool in helping insurance companies calculate rates. Using big data collected from a current risk pool of policyholders that are “sorta like” each other, insurance companies base premiums on a variety of factors.
This way of measuring works on correlations; but as human beings, our minds tend to work on causation. In the case of homeowners insurance, for example, your rates may be higher if you live in an area where your house is at risk for wildfires or other natural disasters. This correlation makes sense to most of us.
But what happens when big data is used to make a correlation between a factor such as low credit score and high risk? Statistics in this instance aren’t taking the “cause” of the low score into consideration. After all, a low credit score can occur for a variety of reasons, including extended joblessness and astronomically high medical bills.
One study concluded that no matter how high your credit score, you’re more likely to get behind on your payments and lose your home if you happen to live in an area with a weak local economy and declining property values. No one truly understands what causes the correlation between low credit score and high risk, and actually there is more evidence showing that it is not discriminatory.
So what message are we sending to consumers if we make judgments based on big data?
As leaders in industries that employ predictive analytics, we need to be vigilant. We must continue to weigh the important differences between correlation and causation and how basing decisions like coverage rates on correlation affects our customers. And we need to continue to consider fairer and more effective ways of utilizing data to set rates.
For instance, at Security First Insurance we have adopted rating factors based on more objective criteria, such as the location of the home and the type of construction, rather than credit score or property risk score.
Other companies are even exploring ways to utilize data-driven analysis of personality. An interesting New York Times post, “Using Algorithms to Determine Character” by Quentin Hardy, explains how the company Upstart has lent millions of dollars in personal loans to folks with “negligible” credit ratings. Since part of the reason those ratings may be that borrowers have briefer employment histories, Upstart considers factors such as the borrowers’ SAT scores and grade point averages as a way of “assessing personality” and basing lending decisions on those assessments.
The insurance industry continues to rely on statistics to more easily — albeit not always fairly — predict the behavior of its customers and to base its coverage rates on the insights the statistics provide. While the topic of correlation vs. causation is a complex one with no definitive solution as yet, we must strive to better understand not only the “what” of correlation, but also the “why” of causation in order to best serve our customers.
Posted in: Home, Insurance, Strategy
Leave a Comment (0) ↓